Data Science Career Advice
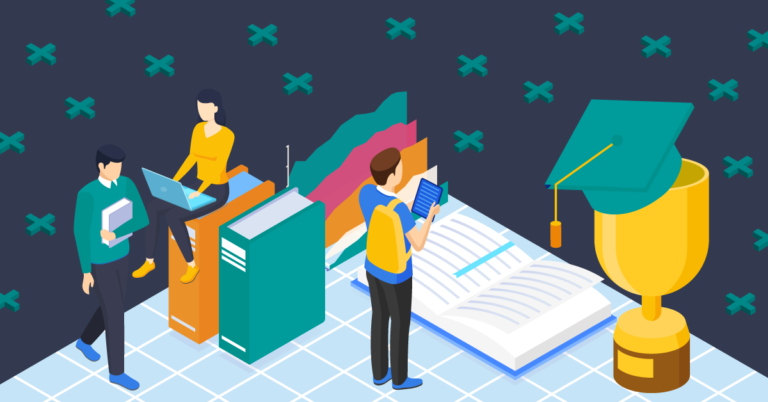

10 Best Data Science Career Advice | Beginners and Professional
Navigating your career path in a relatively new field like Data Science can be daunting. It is more tasking and overwhelming if you are just starting out in the professional world (i.e. fresh out of college).
When people are contemplating a career in Data Science, their minds are often plagued with a series of questions along the lines of:
- ▪ Do I need to learn how to code?
- ▪ Which technique should I focus on?
- ▪ Should I learn statistics first?
- ▪ How about analysis?
- ▪ Does it require a mathematics genius to crack it as a data scientist?
- ▪ Should I learn how to use Python or R? The questions are endless and mind-numbing. However, choosing to pursue a career in data science doesn’t have to be that challenging. This article highlights ten sound pieces of career advice for those looking to venture into data science or professional scientists that are looking to take their careers to new heights. 10 Best Data Science Career Advice: Beginner and Professional From beginners to professionals seeking career insights, these 10 objective pieces of advice can help set you up for long-lasting career success.
1. Decide which area of data science to specialize in
Data Science is an interdisciplinary career field with eight very popular areas that any beginner scientists can explore. A career in each of these areas can turn out lucrative if you pursue it passionately, with the right guidance, and tools.
Depending on your background and prior experience, it is always best for you to choose a definite area of data science and try it out.
Keep in mind, however, that a chosen area of specialization may turn out to be a not-so-great fit and you’d have a need to pivot. That’s absolutely fine. You can confidently try out another area, armed with the knowledge and experiences acquired from your previous try.
It may appear stressful to undergo this “trial by error” run but, trust me, it will provide a directional path for you to follow. That, alone, makes it way easier than just diving into this infinite pool of Data Science, flowing with the waves, and finding no definite career anchor.
But what area should you venture into first, and how does your academic and professional background influence your decision?
A background in mathematics, engineering, and applied statistics, for instance, puts you at an advantage to choose from some of the highest-paying DS career paths. You can explore careers in areas like data mining and statistical analysis, machine learning, and business intelligence.
On the hand, an engineering or other technical background are not prerequisites for choosing a data science career. You can explore opportunities in the growing DS field even with a background in design, communications, architecture, and even general management.
To begin, it would be helpful to seek out the counsel of a career advisor or experienced professional in the field. They could help you assess your strengths as well as the compatibility of your background with the various areas of specialization in data science.
2. Start learning, and don’t stop learning.
Deciding an area of specialization is one step towards becoming a seasoned data science professional. However, merely deciding to follow a path isn’t the same as taking actions that can propel you forward on that path. And the most important action to take in any career is to learn.
Data science has evolved greatly since it spinned out of statistics to encompass non-statistical areas. Interestingly, it is still evolving and you can ride the curves of its evolution by actively learning new ideas and discoveries in your chosen area of specialization.
For beginners, starting with the right data science course can make all the difference. Remember, however, that the choice of course to take will be determined by your chosen specialization.
On SDS Club, you can follow a designed learning path that will take you from beginner to expert on three areas of data science: data visualization, statistical data science, and artificial intelligence. There are several other learning paths that you can explore based on your chosen area of specialization.
Like life itself, building a career in data science requires an insatiable curiosity and drive to continuously gain new insights. Choosing to stop learning is synonymous to putting a titanium ceiling on your progress and tying a 50-pound brick to your growth trend as a professional.
3. Find the right tool or language and master it
The processes that go into working with data are complex. It’s a complete waste of time to try maneuvering Data Science problems using your head. You need to deploy the right tools and machines to solve these problems in a split second.
There are a myriad of data science tools built to solve problems of different types. Companies, both big and small, are in demand of experts that can program and use these tools to solve real-life problems.
If you’ve already chosen an area of specialization and picked up a course from the dozens of available courses on the internet, it is very likely that a tutor has introduced one or two tools that you will need for a career in data science. If choosing the right tool is still a daunting endeavor, you can take a quick look at the most popular data science tools used by professionals of various specialties.
From that list, choose one that is best aligned with your chosen area of specialization and the courses that you are already taking. If you are yet to choose a learning path as suggested in the second advice above, this preferred DS tool can also influence your decision on what course to take.
4. Acquire basic database knowledge and SQL
Every data science professional should have a decent understanding of SQL and databases. This understanding is crucial because you will be dealing with data, which are stored in databases programmed in SQL. Without understanding the infrastructures, data science concepts and processes may fly over your head throughout your career.
5. Infuse practical applications instead of focusing on theory only
Developing a habit or principle for continuous learning may not be enough to set you up for success in a data science career. Practical experiences are more effective than theoretical knowledge.
Therefore, as you study to gain new insights, you have to also cultivate a habit to build machines and infuse the skills that you are acquiring into real-life situations. This doesn’t only improve your understanding of the tools that you are using, but also helps you SHOW potential employers what you’re capable of rather than just TELLING them.
To ensure that you don’t lose your creations and your creative applications of data science techniques in practical ways, you are encouraged to build a personal portfolio to store and showcase these works.
There are several practical applications that you can try, depending on your chosen area of specialization. Here are a few to consider.
- – Creative Data Mining Project Ideas for Any Level
- – 7 Data Analysis Project Ideas to Boost Your Skills
- – 10 Fun Machine Learning Project Ideas for Newbies 6. Use the right resources and seek mentorship The internet is a depot of colossal information. If you run a quick query on Google about high credibility data science resources, you will find hundreds of websites claiming to be the “one-stop shop” for data science information (or something along that line).
While many of these websites share really valuable data science-related insights, they may not be credible sources for new findings and industry-changing insights. You should focus on collecting factual information from credible platforms (e.g. Google Research and several .edu domains).
Navigating the complex internet for verifiable information can be frustrating, especially to a data science beginner. You can avoid most of the frustrations by finding a mentor to guide you.
Data science professionals are very busy people, working 40-50 hours per week. For this reason, they might not be readily available to answer all your questions and teach you all you want to learn. However, whenever they can, they often provide insights that can help you avoid many destabilizing mistakes.
So, while you seek mentors to guide you, endeavor to learn as much as you possibly can on your own. Mentors find it encouraging to guide a self-learner. It’s less teaching and more learning.
7. Join Data Science Communities and Network
Our career aspirations are often fueled by the people we associate ourselves with. Finding yourself in a group of thriving data science professionals will provide the motivation to take the required actions for career success.
Other than motivation and elevated aspirations, information is your lifeblood as a data science professional. Information flux within data science communities (such as the DMC) is super fast. New industry findings, tools, hacks, etc. spread inside the community almost immediately after it’s discovered. Meanwhile, it could take a while before that information reaches other professionals that are outside the communities.
One of the best things you can do for your data science career is to find appropriate (physical and virtual) communities to join. And merely joining is not enough!
You need to voluntarily engage with these communities, network with other members, and share opportunities among yourselves. Who knows, your next big opportunity could come from a simple conversation with a few community members.
8. Build and/or deploy models to prove proficiency
Programming algorithms and building models in data science are respectable achievements that are reserved for plausible data scientists. The same goes for deploying these models by developers.
Course curators and tutors seldom include model building and deployment in their beginner courses. This is for a good reason though. Building algorithms and models can be a frustrating project to pursue, even for seasoned professionals. Introducing a beginner to this intensive process may harm their enthusiasm and interest in data science careers.
To set you aside from beginners and position yourself as a professional in any given area of data science, you have to work your way into this group of plausible data scientists. You can do this by building a model of your own or learning how to deploy existing ML models.
However, ensure to keep these achievements in your portfolio, no matter how little they are.
9. Develop important soft skills
Building a successful career requires more than being the best data analyst or building and deploying the most insanely cool models. No doubt, being the best at what you do makes you indispensable. However, being indispensable doesn’t translate to a successful data science career.
Along with being the best in your area of specialization, you have to develop the necessary soft skills to thrive in a professional environment. These skills include effective communication, emotional intelligence, negotiation, conflict resolution, etc.
These skills will help you manage the people and relationships around you, and understand how you can work with each person to contribute immensely to the growth of the organization and involved persons. Your career will grow faster if you can build and maintain a solid interpersonal relationships.
10. Build your resume
Ever since Leonardo da Vinci created the first resume 500 years ago, resumes are our first point of contact professionally. They are representations of us, our experiences, and our abilities. There’s a limit to the number of faces you’d meet and hands you’d shake but your resume can travel way farther.
With LinkedIn serving as the digital resume for many professionals, that could be a good place to start building this creative representation of you.
As a data science professional, your LinkedIn profile should reflect your abilities, qualifications, experiences, and links to your portfolio for potential employers and clients to preview. You can create a traditional resume by customizing any of the one hundred dozen resume templates on the internet.
Sadly, you may present an empty resume if you don’t have the experiences, qualifications, skills, and proven practical applications to show. And it is unethical to lie in your resume. In some countries, it is outright illegal.
So, to build a solid resume, you should follow the first 9 pieces of advice included in this article and document your achievements, experiences, and earned certifications accordingly.
On a final note…
Thriving in an evolving industry is challenging and exciting. There’s so much to learn and the potential is enormous. Data science presents this interesting mix of excitement and challenge to people looking to pursue a career in it.
However, just jumping into the ocean of data science-related professions can be counterproductive. As you set your foot into the said ocean, ensure to set sail in a specific direction.
Then, learn your way upward. Keep an open mind and be willing to pivot if you think you have a better chance in a different area of data science.
Whatever area of specialization you choose to follow through, you need a robust network of like-minded professionals, as well as a good source of industry insights.
Stay up to date with the SDSClub and the DMC https://discord.gg/gZq2538tCt to capture new insights, network, and continue learning to help achieve your goals!