Unlocking the AI Potential: Role of Machine Learning Engineer in the New Era
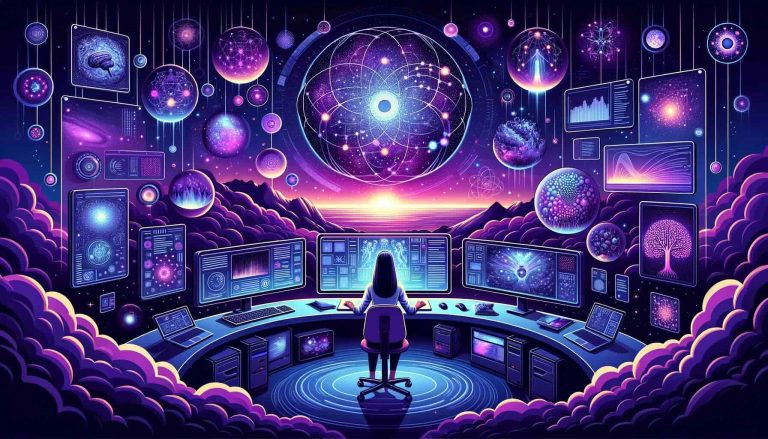
Artificial Intelligence in the New Era: The Rise of Generative Machine Learning
Introduction to the New Age Machine Learning Engineer
A new breed of professionals has emerged in the rapidly evolving landscape of artificial intelligence: the New Age Machine Learning Engineer. While rooted in traditional machine learning practices, this role has evolved to address the unique challenges and opportunities of generative AI.
Traditional vs. New Age Machine Learning Engineer
The Pine Cone AI Transformation Summit Insight
The Pine Cone AI Transformation Summit recently introduced the concept of the New Age Machine Learning Engineer. This term, while novel, resonated with many in the industry, particularly those who have been navigating the shifting terrains of AI projects in recent months. The summit highlighted the distinction between traditional machine learning engineers, who primarily focus on classical algorithms and data-driven models, and their new-age counterparts, who are deeply entrenched in the world of generative AI.
The Evolution of Machine Learning Projects
Historically, machine learning projects revolved around training algorithms using specific datasets to solve problems. The goal was to find the optimal algorithm and dataset combination to build a robust machine-learning solution. However, the approach has shifted with the advent of pre-trained generative AI models. Instead of training models from scratch, engineers now interact with pre-existing models through APIs, leveraging their capabilities while adding specific customizations to suit particular applications. This evolution signifies a move from purely data-driven projects to a more application-driven approach, where the integration of various tools and platforms becomes paramount.
The Role and Impact of Generative AI
The Shift from Traditional to Generative AI
Generative AI represents a significant departure from traditional machine learning paradigms. While traditional models are trained to make predictions based on input data, generative models are designed to generate new data that mirrors the input data’s characteristics. This capability has opened up a plethora of applications, from content creation to data augmentation. However, the shift also brings challenges. Interacting with pre-trained models requires a different skill set, primarily centered around integrating these models into broader applications, often through APIs.
The Importance of Software Engineering Skills in AI
The rise of generative AI has underscored the need for robust software engineering skills among machine learning engineers. In traditional machine learning, the focus was primarily on selecting and tuning algorithms. However, with generative AI, the emphasis is on building applications. For instance, the process of retrieval augmented generation involves using vector databases to store data, retrieving it, and then passing it as input to large language models. This process necessitates the integration of various services, from vector databases to language model APIs, and potentially building out entire applications with multiple moving parts. As such, proficiency in software engineering, particularly in building and integrating APIs, has become indispensable in generative AI.
Challenges and Innovations in Generative AI Engineering
Generative AI, while a groundbreaking advancement in artificial intelligence, presents distinct differences and challenges compared to traditional machine learning approaches. These differences are primarily rooted in the software engineering requirements and the unique mindset needed to effectively harness the power of generative models.
The Software Engineering Aspect
The Need for More Software Engineering Skills
Generative AI projects demand a significant enhancement in software engineering skills compared to traditional machine learning endeavors. Historically, machine learning engineers focused on algorithm selection and data processing. However, with the rise of generative AI, there’s a shift towards building intricate applications. These applications often involve interacting with pre-trained models via APIs, necessitating a deep understanding of software integration, API interactions, and application development. The transition from algorithm-centric tasks to application-driven projects underscores the importance of robust software engineering capabilities.
The Process of Retrieval Augmented Generation
A pivotal technique in the realm of generative AI is the process of retrieval augmented generation. This method involves storing data in vector databases, retrieving specific data segments, and then using them as inputs for large language models. The entire process is more intricate than traditional machine learning tasks. It requires seamless integration of various services, from the vector database to the language model API. The primary challenge lies in ensuring that these services communicate effectively, retrieve accurate data, and generate relevant outputs. Mastery of this process is crucial for building efficient generative AI applications.
The Data Scientist Mindset in Generative AI
The Non-deterministic Nature of Pre-trained Language Models
One of the fundamental challenges with generative AI is the non-deterministic behavior of pre-trained language models. Unlike traditional software engineering, where specific inputs yield predictable outputs, generative models can produce varied results for the same input. This unpredictability stems from the inherent design of these models, which are trained to generate data rather than predict based on existing data. For instance, even with a fixed temperature setting, the outputs can vary based on subtle changes in user input. This non-deterministic nature demands a different approach that is familiar to data scientists but might be foreign to traditional software engineers.
The Importance of Context and Data in AI Applications
Generative AI thrives on context. While pre-trained models are powerful, they lack specificity for unique business problems. To harness their full potential, providing them with the proper context is essential, often achieved through techniques like retrieval augmented generation. The challenge lies in ensuring that the context is accurate and relevant. For instance, two slightly different user queries might retrieve different data segments from a vector database, leading to varied outputs. Ensuring that the model receives the correct context is paramount. Additionally, understanding the nuances of structured or unstructured data and processing it effectively is crucial for the success of generative AI applications.
Applying Generative AI Tools in the Expanding Business Landscape
The integration of Generative AI into the business domain has opened up a plethora of opportunities and challenges. As companies navigate this new frontier, understanding its practical applications, the role of the New Age Machine Learning Engineer, and the future interplay between generative and traditional approaches becomes crucial.
Practical Applications and Considerations
The Impact of AI on Customer Experience
Generative AI has begun to significantly influence customer experience, especially with the advent of AI-driven chatbots and support systems. These tools, powered by large language models, can provide instant responses to customer queries. However, the accuracy of these responses is paramount. For instance, a query about a product’s return policy must yield a precise answer, as inaccuracies can lead to legal implications and diminished customer trust. Thus, while generative AI can enhance customer interactions, ensuring the reliability of its outputs is essential.
The Role of the New Age Machine Learning Engineer in Business
The New Age Machine Learning Engineer plays a pivotal role in harnessing the power of generative AI for business applications. Beyond traditional machine learning tasks, they are responsible for integrating pre-trained models into broader applications, often through APIs. Their expertise lies in understanding the AI models and building applications that can interact effectively with these models. They bridge the gap between generative AI capabilities and specific business needs, ensuring that AI tools are tailored to address unique business challenges and add tangible value.
The Future of Generative AI and Traditional Approaches
The Relevance of Traditional Machine Learning Tools
While generative AI makes headlines, traditional machine-learning tools remain highly relevant. These tools, encompassing classical algorithms and data-driven models, continue to address various tasks and challenges. For many businesses, traditional machine-learning approaches are still novel and unexplored. As such, while it’s essential to keep an eye on the advancements in generative AI, it’s equally crucial to recognize the value and potential of traditional tools. They remain foundational in the AI toolkit and continue to offer solutions to diverse business problems.
The Role of Data Alchemy and the Alchemy Codex in AI
Data Alchemy, with its Alchemy Codex, plays a significant role in the broader AI landscape. The Alchemy Codex serves as a comprehensive set of tools, skills, and workflows essential for transforming raw data into actionable insights. Whether one aims to be a data scientist, a traditional machine learning engineer, or a New Age machine learning engineer, the fundamentals outlined in the Alchemy Codex are indispensable. It emphasizes the core principle of turning data into valuable information, irrespective of the specific AI tool or approach in use. As the AI domain continues to evolve, resources like the Alchemy Codex provide a solid foundation, ensuring that professionals are equipped with the essential skills to navigate the ever-changing landscape.